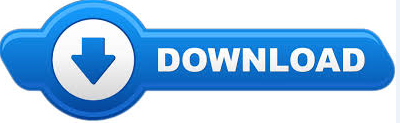
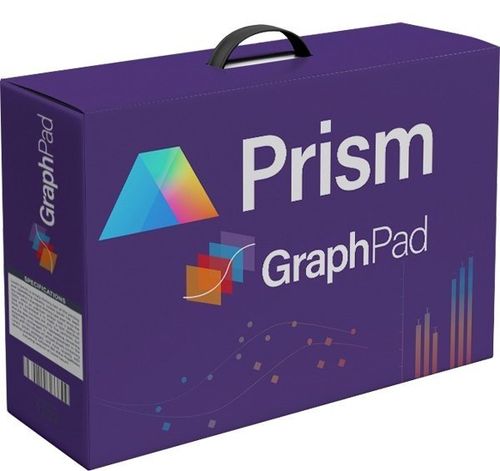
You are not interested in variation among those particular participants, but want to know about variation among participants in general. When Prism does mixed-model analysis of repeated measures data, it assumes that the main factors (defined by the data set columns in one-way, and by data set columns and rows in two- and three-way) are fixed, but that subjects (or participants, or runs.) are random. The model is mixed because there are both fixed and random factors. In Prism, ANOVA treats all factors, including participant or block, as fixed factors.Īs the name suggests, the mixed effects model approach fits a model to the data. With repeated measures ANOVA, one of those components is variation among participants or blocks.

Prism uses the mixed effects model in only this one context. Prism uses a mixed effects model approach that gives the same results as repeated measures ANOVA if there are no missing values, and comparable results when there are missing values. Prism 8 fits the mixed effects model for repeated measures data. Because of this versatility, the mixed effects model approach (in general) is not for beginners. Many books have been written on the mixed effects model. The mixed effects model approach is very general and can be used (in general, not in Prism) to analyze a wide variety of experimental designs. Fitting a mixed effects model - the big picture Prism offers fitting a mixed effects model to analyze repeated measures data with missing values. This is not a preferred method, and is not offered by Prism. The only way to overcome this (using ANOVA) would be to impute what the values of the missing values probably were and then analyze without any missing values, correcting the results (reducing df) to account for the imputing. If a value is missing for one partiicpant or animal, you'd need to ignore all data for that participant or animal. Repeated measures ANOVA calculations require complete data.

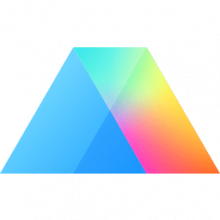
The problem: Repeated measures ANOVA cannot handle missing values
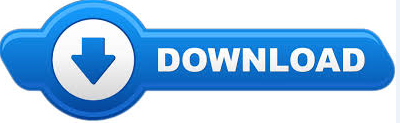